Florence-2: Advancing a Unified Representation for a Variety of Vision Tasks
Florence-2 represents a significant leap forward in the field of artificial intelligence, particularly in computer vision. As a successor to Florence, this advanced model brings a unified approach to handling multiple vision tasks. By integrating various learning techniques and leveraging extensive datasets, Florence-2 enhances its ability to process and interpret visual data efficiently. Its development aims to improve applications in areas such as object recognition, captioning, and image retrieval, establishing a new benchmark for general-purpose vision models.
The Evolution of Florence-2
The development of Florence-2 was driven by the need for a more versatile and efficient vision model capable of performing diverse tasks with high accuracy. Unlike traditional models that specialize in a narrow set of vision functions, Florence-2 employs a unified representation approach. This means that a single model can seamlessly adapt to different vision-based tasks instead of requiring multiple models for different applications.
Florence-2 builds upon its predecessor by incorporating more extensive datasets and leveraging advanced self-supervised learning techniques. The model’s architecture has been refined to improve both accuracy and generalization, allowing it to excel in tasks such as image classification, segmentation, and object detection.
Technical Innovations in Florence-2
Several key innovations make Florence-2 a standout model in the field:
- Unified Representation Learning: By developing a shared representation that works across multiple vision tasks, Florence-2 eliminates the need for task-specific models, improving efficiency and scalability.
- Enhanced Multimodal Capabilities: Florence-2 is designed to process and understand both images and text in a cohesive manner, making it suitable for applications such as text-to-image retrieval and image captioning.
- Improved Generalization: Leveraging vast and diverse datasets, Florence-2 demonstrates superior generalization across different datasets and use cases, reducing the risk of overfitting and enhancing performance on unseen data.
- Self-Supervised Learning: The model efficiently learns representations without requiring manually labeled data, making it highly adaptable to various real-world scenarios.
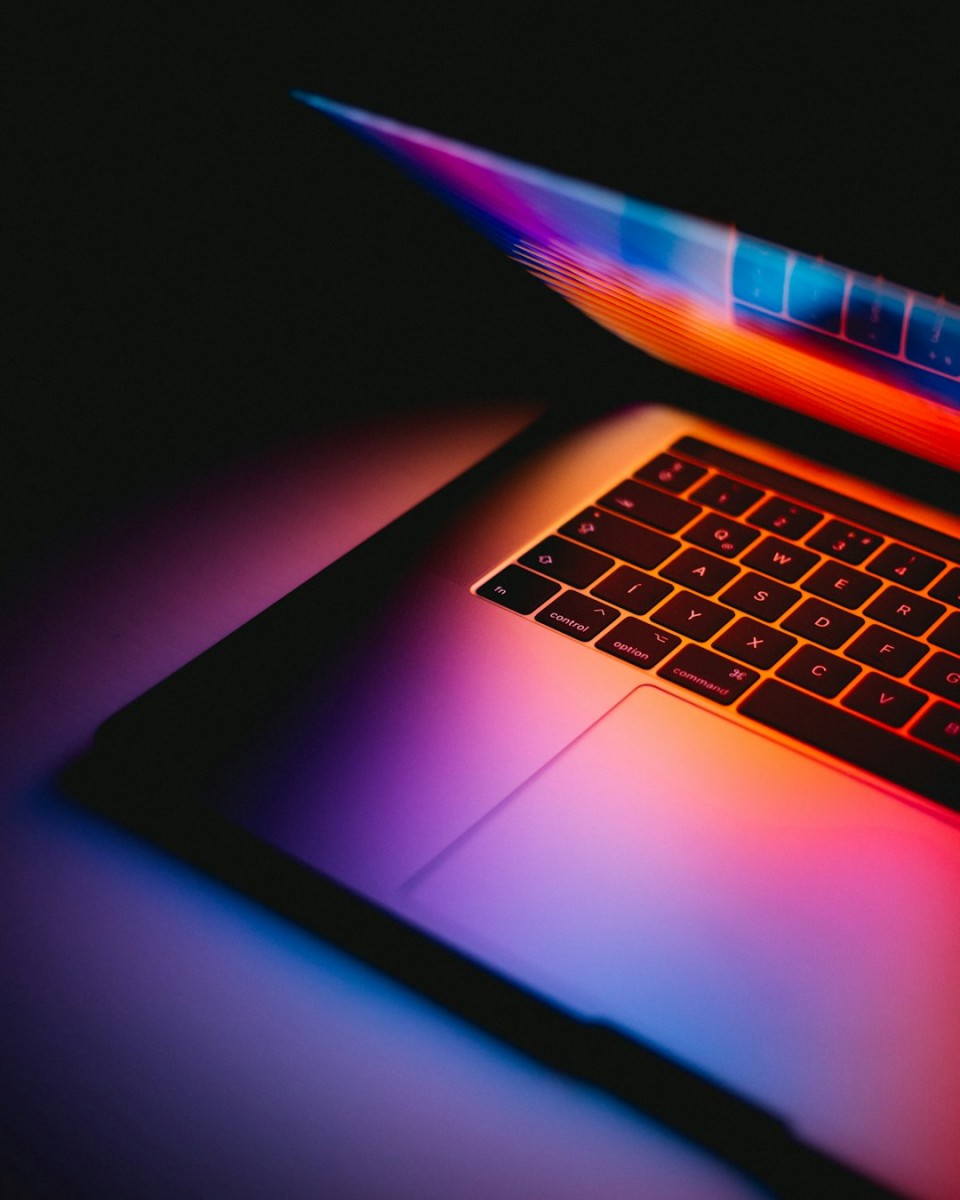
Application Areas of Florence-2
Florence-2 is designed to be applied in a wide range of industries and sectors. Some of the most notable applications include:
- Healthcare: Medical image analysis and diagnostics benefit from Florence-2’s ability to identify patterns in complex imaging data.
- E-commerce: Retailers can use Florence-2 for product categorization, visual search, and recommendation systems.
- Autonomous Vehicles: Enhanced object detection and segmentation capabilities help improve the accuracy and safety of self-driving cars.
- Surveillance and Security: The model is useful for facial recognition, anomaly detection, and threat identification in security applications.
- Creative Content Generation: Florence-2 plays a role in areas such as AI-generated art, automated video captioning, and interactive media.
Advantages Over Previous Models
Florence-2 surpasses previous vision models in several ways:
- Higher Efficiency: With its unified representation structure, it reduces computational cost and training time.
- Better Performance Across Tasks: Unlike specialized models, Florence-2 can perform multiple tasks with a single architecture, maintaining high accuracy across different domains.
- Scalability: The model’s ability to generalize enables it to be deployed in various real-world applications without significant modifications.
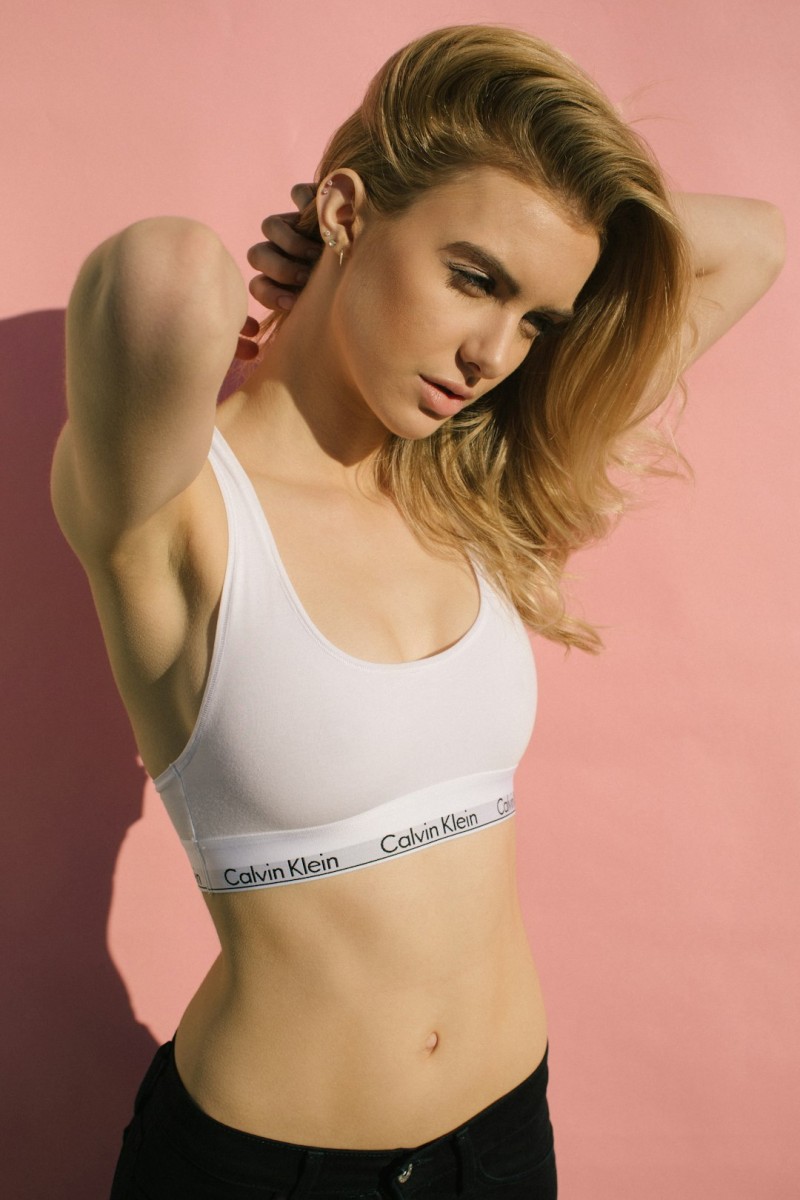
Challenges and Limitations
While Florence-2 brings numerous advancements, it also faces challenges such as:
- Data Bias: The model’s performance is influenced by the datasets used for training, making it susceptible to biases.
- Computational Demands: Running Florence-2 requires substantial computational resources, which may not be accessible to all users.
- Interpretability: Understanding and explaining Florence-2’s decision-making process remains a challenge, as is common with deep learning models.
Future Prospects
As AI research continues to evolve, Florence-2 serves as a strong foundation for future developments in vision models. Researchers will likely focus on improving its interpretability, reducing computational demands, and minimizing biases. Additionally, integrating Florence-2 with larger multimodal AI systems could lead to more advanced capabilities, such as enhanced natural language understanding combined with computer vision.
Frequently Asked Questions (FAQ)
What makes Florence-2 different from other vision models?
Florence-2 employs a unified representation approach, allowing it to handle multiple vision tasks efficiently with a single model, unlike traditional models that focus only on specific tasks.
What are some real-world applications of Florence-2?
Florence-2 is used in industries such as healthcare (medical imaging), e-commerce (visual search), autonomous vehicles (object detection), security (facial recognition), and creative content generation.
Does Florence-2 require labeled data for training?
No, Florence-2 leverages self-supervised learning, which enables it to learn patterns from large datasets without extensive manual labeling.
What are some limitations of Florence-2?
Challenges include potential data bias, high computational demands, and difficulties in interpretability inherent to deep learning models.
How does Florence-2 contribute to AI research?
Florence-2 advances unified vision models by improving generalization, multimodal learning, and efficiency, paving the way for future AI developments in computer vision.